Summary
Representatives in the Schuylkill Delegation presented a retirement citation to Lynn Sabol, who has dedicated 45 years to education, with 15 of those years as principal at Nativity BVM High School
Source: The Republican Herald
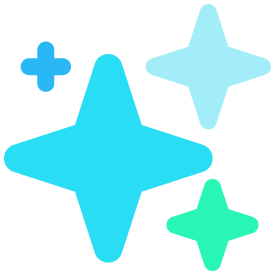
AI News Q&A (Free Content)
Q1: What are the primary factors influencing retirement decisions in modern societies, and how has the concept of retirement evolved historically?
A1: Retirement decisions are influenced by eligibility for pension benefits, health status, and legal requirements. Historically, retirement was rare due to low life expectancy and lack of social security, with most people working until death. The introduction of state pensions in the late 19th and early 20th centuries, starting with Germany in 1889, gradually made retirement a right for workers in many countries. Today, people may choose to retire fully, semi-retire, or delay retirement, with developed nations offering structured pension support, while in poorer regions, support often comes from family.
Q2: How does the statutory retirement age vary globally, and what implications does this have for senior health and economic security?
A2: The statutory retirement age differs by country, typically ranging between 60 and 67 years. This age often marks eligibility for government or employer pensions. Variations reflect economic, demographic, and political factors, affecting when individuals can access retirement benefits. Early or delayed retirement can impact seniors’ health outcomes and economic security, with earlier retirement sometimes linked to lower income but potentially better health, while delayed retirement may offer higher pension benefits but can also present workplace challenges for older adults.
Q3: What recent technological innovations are being implemented to improve the health and wellness of senior citizens in post-retirement living environments?
A3: Recent innovations include automated vision-based wellness analysis systems in elderly care centers, which use deep neural networks to monitor seniors' physical and mental well-being via video data. These systems provide caregivers with wellness metrics and health patterns, enabling more tailored caregiving strategies. Additionally, mobile-health solutions like ACTA offer integrated cognitive training with adaptive machine learning, supporting independent and active aging for seniors with mild cognitive impairments.
Q4: What are the main challenges associated with digital technology adoption among retirees, particularly in the context of health and social inclusion?
A4: Many retirees face difficulties adapting to digital technologies, particularly mobile-based health apps introduced during the COVID-19 pandemic. Barriers include unfamiliarity with user interfaces and lack of digital literacy, leading to reduced mobility and increased social isolation. These challenges can negatively affect both physical and mental health, as retirees may avoid essential activities requiring digital verification, such as travel or accessing health services.
Q5: What does recent research reveal about the impact of delayed retirement on senior health across different demographic groups?
A5: A recent study compared the effects of late retirement on health across gender and occupational groups. Findings showed that while delayed retirement can provide financial benefits and increased pension security, the health impacts vary. Some groups experienced improved mental engagement and well-being, while others faced increased physical or psychological stress. The research underscores the need for individualized approaches when considering retirement timing.
Q6: How can the use of advanced statistical models improve the accuracy and fairness of retirement benefits for seniors?
A6: Advanced models like the gamma-Gompertz-Makeham (GGM) are being used to more accurately estimate life expectancy and mortality rates for retirement benefit calculations. Research in Brazil indicated that official life tables, when right-censored, underestimated life expectancy for those delaying retirement, potentially reducing their retirement income. GGM models provided more stable and realistic estimations, supporting fairer benefit distributions and improved long-term financial planning for retirees.
Q7: How are machine learning and natural language processing (NLP) models being used to assess mental health in retirement communities, and what are their limitations?
A7: Machine learning and NLP models are increasingly used to predict depression and monitor mental health among retirees. A recent study found that NLP-based depression models trained on younger populations performed well on senior data, with only modest accuracy degradation. However, model performance was higher among seniors with consistent health states, highlighting the need for demographic-specific tuning. Limitations include generalizability across diverse age groups and ensuring privacy and ethical data use.
References:
- Retirement - Wikipedia: https://en.wikipedia.org/wiki/Retirement
- Retirement age - Wikipedia: https://en.wikipedia.org/wiki/Retirement_age